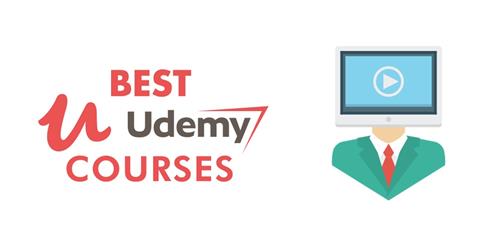
Free Download Akka Remoting and Clustering with Scala – Rock the JVM
Last updated 4/2022
Created by Daniel Ciocîrlan
MP4 | Video: h264, 1280x720 | Audio: AAC, 44.1 KHz, 2 Ch
Genre: eLearning | Language: English + srt | Duration: 20 Lectures ( 6h 50m ) | Size: 4.23 GB
Полная новость
Last updated 4/2022
Created by Daniel Ciocîrlan
MP4 | Video: h264, 1280x720 | Audio: AAC, 44.1 KHz, 2 Ch
Genre: eLearning | Language: English + srt | Duration: 20 Lectures ( 6h 50m ) | Size: 4.23 GB
For Scala and Akka developers: write reactive distributed systems with remote actors, Akka Clustering and Scala
Free Download What you'll learn:
Created by Daniel Ciocîrlan
MP4 | Video: h264, 1280x720 | Audio: AAC, 44.1 KHz, 2 Ch
Genre: eLearning | Language: English + srt | Duration: 20 Lectures ( 6h 50m ) | Size: 4.23 GB
For Scala and Akka developers: write reactive distributed systems with remote actors, Akka Clustering and Scala
Free Download What you'll learn:
Полная новость
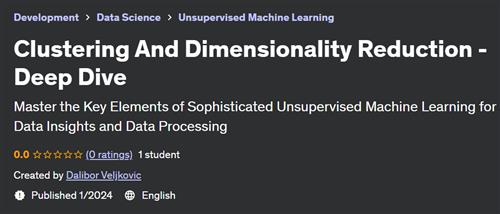
Free Download Clustering And Dimensionality Reduction – Deep Dive
Published 1/2024
Created by Dalibor Veljkovic
MP4 | Video: h264, 1280x720 | Audio: AAC, 44.1 KHz, 2 Ch
Genre: eLearning | Language: English | Duration: 203 Lectures ( 27h 40m ) | Size: 10.4 GB
Полная новость
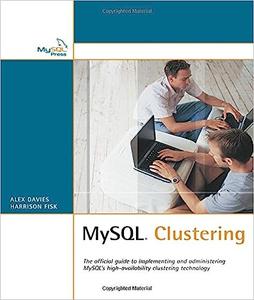
Free Download Harrison Fisk, "MySQL Clustering"
English | 2006 | pages: 217 | ISBN: 0672328550 | PDF | 1,3 mb
Clustering is the final component of MySQL that makes it truly enterprise-level and able to compete fully with proprietary databases such as Oracle and Microsoft SQL Server. The increased number of high-demand, high-productivity corporations and institutions choosing MySQL, including MIT, the Department of Homeland Security, NASA, and Nokia, to name a few, need the benefit of clustering databases for high performance and scalability.
Полная новость
- Книги
- 25-03-2023, 14:52
- 105
- 0
- voska89
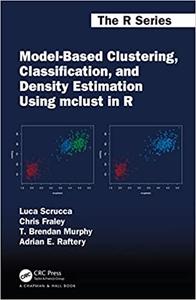
Free Download Model-Based Clustering, Classification, and Density Estimation Using mclust in R
by Luca Scrucca
English | 2023 | ISBN: 1032234962| 269 pages | True PDF | 28.26 MB
Полная новость
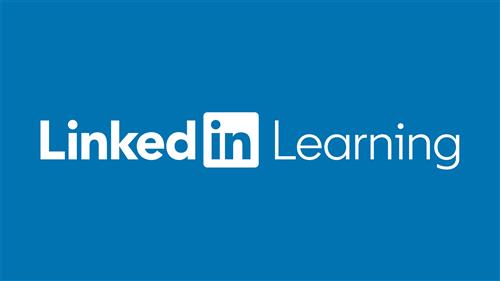
Unsupervised learning is a type of machine learning where algorithms parse unlabeled data. The focus is not on sorting data into known categories but uncovering hidden patterns. Unsupervised learning plays a big role in modern marketing segmentation, fraud detection, and market basket analysis. This course shows how to use leading machine-learning techniques—cluster analysis, anomaly detection, and association rules—to get accurate, meaningful results from big data.
Instructor Keith McCormick reviews the most common clustering algorithms: hierarchical, k-means, BIRCH, and self-organizing maps (SOM). He uses the same algorithms for anomaly detection, with additional specialized functions available in IBM SPSS Modeler. He closes the course with a review of association rules and sequence detection, and also provides some resources for learning more.
All exercises are demonstrated in IBM SPSS Modeler and IBM SPSS Statistics, but the emphasis is on concepts, not the mechanics of the software.
Duration: 3h 22m | .MP4 1280x720, 30 fps(r) | AAC, 48000 Hz, 2ch | 532 MB
Полная новость
- Книги
- 25-01-2023, 06:09
- 114
- 0
- voska89
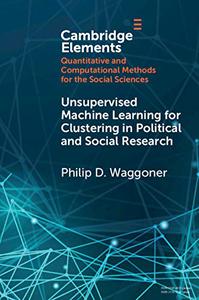
Unsupervised Machine Learning for Clustering in Political and Social Research
English | 2020 | ISBN: 110879338X | 70 Pages | PDF (True) | 10 MB
In the age of data-driven problem-solving, applying sophisticated computational tools for explaining substantive phenomena is a valuable skill. Yet, application of methods assumes an understanding of the data, structure, and patterns that influence the broader research program. This Element offers researchers and teachers an introduction to clustering, which is a prominent class of unsupervised machine learning for exploring and understanding latent, non-random structure in data. A suite of widely used clustering techniques is covered in this Element, in addition to R code and real data to facilitate interaction with the concepts. Upon setting the stage for clustering, the following algorithms are detailed: agglomerative hierarchical clustering, k-means clustering, Gaussian mixture models, and at a higher-level, fuzzy C-means clustering, DBSCAN, and partitioning around medoids (k-medoids) clustering.
Полная новость